Since AI is only as powerful as the quality and quantity data informing it, AI’s evolution from a nice-to-have to table stakes means it’s never been more crucial to have accurately labelled and unbiased datasets feeding your artificial intelligence models and algorithms.
But there’s still a big disconnect at many brands: they know robust data pipelines and AI operations are key that to realizing business value from their AI initiatives, but they lack the manpower or strategy (or both) to scale and sustain data and AI operations. That means they’re not capitalizing on all that data’s power to create AI models that automate customer journeys or anticipate customer need.
More than half (51%) of decision makers say data accuracy is critical to the success of AI, yet only 6% say they’ve achieved data accuracy higher than 90%, according to a 2022 report by Appen that polled more than 500 decision makers in the United States, United Kingdom, Ireland and Germany.
The contact center houses so much data that can be leveraged to develop AI models specific customer support processes -- are you harnessing its full potential? It could be time to rethink your approach, starting with increasing your investment in data annotation.
Data and AI ops are business necessities
The strategic nature of artificial intelligence requires a thoughtful investment in data and AI operations. The days of relying upon crowdsourced services can undermine your business objective and often results in rework or ineffective applications of AI.
In the Appen report, 51% of respondents said data accuracy is critical to the way they use AI, but 39% said they struggle with a lack of qualified people or technical resources. That’s a major barrier.
The old way of hiring data annotators – crowdsourcing and paying them per annotation – no longer makes sense. That outdated approach only incentivizes annotators to work quickly, prompting them to rush and comprise the quality of your annotations along the way.
With data annotation, quality is as important as quantity. So focus on finding high-quality annotators who will perform the job with a high accuracy rate. Seek out annotators who possess vertical-specific expertise that’s relevant to your business. The more specialized your data is, the more nuanced expertise your annotators should have.
Prioritize the integrity of your model
Being more discerning about the annotators you hire will help keep the integrity of your model, but there are a few other things you can do to ensure your annotation methods pay off over the long run.
There’s nothing worse than realizing you left valuable data out of the equation at the outset and finding that you’ve got to restart your modeling from scratch. That’s why its critical to have your model fleshed out first; you need to know what you’re looking for and take stock of all the available data you may have – before you ever hand it over to your annotators.
Taking time to front-load the process in this way, casting a wider net from the beginning when it comes to your data, can save time-consuming and expensive headaches down the line.
Work with an expert partner
There’s never been a better time to rethink your approach to data annotation and, with AI penetrating more of the contact center space, there’s no time to waste. For many, changing the way you think about annotation could seem daunting, but you don’t have to do it alone.
Working an expert partner brings many advantages. A strong partner can help you upgrade the performance of your annotators, or provide highly skilled annotators of their own, as well as guide you through the modeling process.
An outside partner can also take a more a holistic view of your business and identify ways to connect your AI with customer experience (CX) operations – bringing the perspective you need to elevate your CX through design, training, and continual optimization.
To get the most out of AI, invest in high-quality data annotation
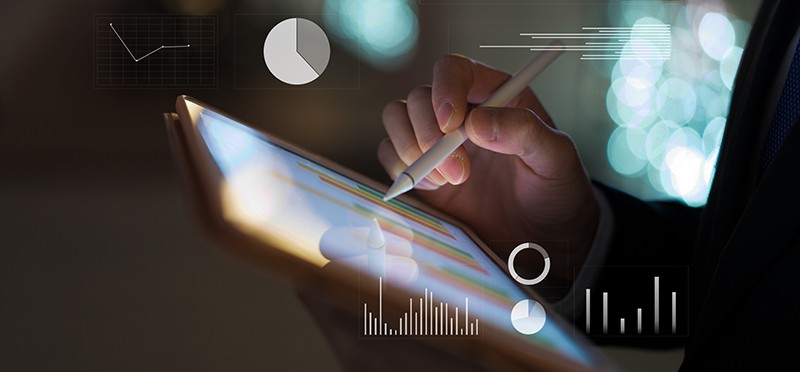