Despite a growth in digital and self-service customer service channels, customers still use the standard, live-support channels—73 percent use the phone, 68 percent use email, and 58 percent use chat, according to Forrester. And that is a good thing, because within these channels lies the opportunity for companies to leverage powerful analytics to identify new or improve self-help offerings; improve products, processes and services in order to reduce customer calls and inquiries; and enhance the overall customer experience when engaging with your brand.
Recently, we worked with a software provider to identify how best to allocate certain product and customer service investments. Every year, the client sets aside budget to make various product improvements that will reduce call volume to its contact centers, but this time it was looking for a more data-driven approach.
The client’s goal is always the same—to allocate investments to the most beneficial activities that drive down call volume and increase customer satisfaction. Part of this year’s corporate challenge was to find a solution that would provide continuous insights into how to make ongoing gains, rather than just a one-time fix.
Working with the client, we examined the assorted data sources that the company maintains, including several structured and unstructured data sources containing over 3 million customer cases. Within the structured data set, there were more than 300 call topics and 1,300 call reasons captured by associates, making it hard to pinpoint where to investigate and invest. In addition to finding duplicate or very similar topics and reasons, the descriptions did not adequately explain what the customer problem was or where to focus improvement efforts.
Through guided text mining of the customer interactions captured in associate notes, we developed a new topic and reason categorization with more actionable insights in mind. The process for building this new categorization included collaborative meetings with the client to select a few major topics to explore, review of more than 500 cases to provide the right inputs for the guided text mining and querying, a QC process to ensure text mining efforts and new categories captured the vast majority of customer interactions, and then rolling out this proof of concept to the full data set of interactions.
The new structure for identifying customer issues was reduced to just 50 categories and 180 sub-categories, covering more than 80 percent of all of customer contacts. The new categories honed in on the issues from the customer point of view and better described the situations the customer was facing. Very quickly, the client could identify easy product fixes that affected a large volume of calls, as well as a number of “How do I…” and “Where do I find…” type calls related to user status, scheduling, or automated processes and confirmations that could be handled through enhanced self-help.
In addition, the client leveraged TeleTech Insights cloud-based analytic platform and automated data visualization dashboards to identify the next best places for immediate investment, the relative volume of customer issues in these areas, and to track the progress of any initiatives.
Dashboards also allowed for the tracking of other key customer metrics. For example, a simple question on Net Promoter Score (NPS) led to a separate dashboard view where the client could better track NPS by region, segment, and other attributes. And this customer satisfaction information was integrated into call activity and volume dashboards to provide a more comprehensive view of the customer relationship.
Together with the client, we developed business cases to justify product improvement investments in key categories, as well as the funding of enhanced self-help options.
In total, the gain from using this advanced approach to issue categorization is estimated to reduce call volume by more than 30 percent over three years, representing tens of millions of dollars saved. Plus, the client expects to observe better customer experiences and higher customer satisfaction by enabling customers to solve many issues on their own.
What’s next? We developed an automated hot topic / emerging topic alert system to capture new issues as they arise. This alert system will ensure new customer concerns can be captured earlier and addressed before the volume of calls increases. We are also working with the client to identify other data sources (e.g., user community feedback, employee product feedback) to enhance and expand the categorization structure and further improve and justify investment decisions. Finally, we are very excited that the client has suggested rolling out this new approach company-wide to all their divisions—a great validation of our work.
Text Mining for Data Gold in the Contact Center: A Client Story
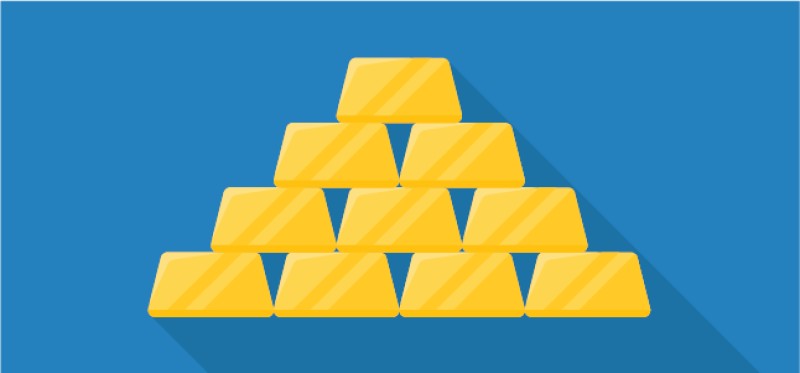