When it comes to Big Data strategy, the telecom industry has an advantage over others due to the sheer breadth and depth of data it collects in the course of normal business. For example, an operator serving 8 million prepaid mobile subscribers generates around 30 million Call Data Records (CDRs) daily, equaling 11 billion records annually. If the same operator also provides postpaid and fixed lines services, then there is even more volume and variety of data at the ready.
Data collection, storage, and dissemination are built into the everyday operations of most communications companies. And a majority already staff many data scientists and analysts to cull the information into insight. But few are using that valuable information to its fullest potential.
There are many types of data and information that can be optimized with a sound Big Data strategy (see Figure 1). So what should an operator do with all this information? Looking at Big Data through a customer lens offers three strong opportunities: improve marketing effectiveness, enhance customers' experiences, and develop new sources of revenue.
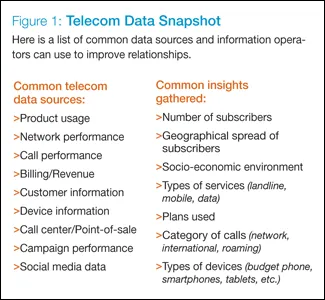
Marketing effectiveness: Big Data helps telecom operators improve their marketing effectiveness in a number of ways. Personalized, relevant actions can be taken based on real-time information without the need to wait for data extraction or manual data mining. Targeted campaign performance can be streamlined with the help of advanced analytical models, such as churn prediction or next best offer. These models provide valuable proactive customer insights that can be utilized for cross-sell, up-sell, or retention campaigns, based on analysis of the customer's preferences and behavior. And Big Data helps to reveal preferred channels for targeting customers, which enhance response rates and have an incremental positive impact on the overall campaign performance and efficiency.
Customer experience: Big Data can help gather real-time customer satisfaction information through social media listening or Voice of the Customer analysis, for example. This can be done at the customer level in order to address a specific customer complaint, or at the entire subscriber base level to detect macro customer themes. Operators can get feedback about a new product launch, a major brand announcement, or a simple network issue, for example. Big Data also facilitates the transition toward better self-service systems. Furthermore, analytical models, such as segmentation, can also improve customer experience by identifying the most valuable customers who would benefit from dedicated treatment and better services.
Revenue impact: Once mastered by the telecom operator, Big Data can be utilized to create new products. With so much insight at their fingertips, operators can use the knowledge they possess to help their B2B clients address their Big Data challenges. Or they can enter into partnerships with third parties to share aggregate or subscriber-level data. For example, retailers may be interested in the operators' customers' location-based data in order to target them with specific promotions when they are close to a store. And over-the-top (OTT) players like Google, Skype, and Netflix may be interested in behavioral data to target consumers with specific content.
Such opportunities hold promise, but keep in mind there are many legal, ethical, and reputational effects of Big Data initiatives. They must be coupled with a serious reflection about the different ways to acquire and share data, or entice the customer to allow such scenarios. This is where the customer lens is vital to success.
ROI of Big Data
Peppers & Rogers Group recently worked with a mobile operator to improve its marketing effectiveness and ROI. The client did not have detailed data for its mobile subscribers, and the average time to market for a targeted marketing campaign was one month. It was simply not interacting with customers fast enough or with the right messages.
The team constructed a data mart containing a summary of all detailed international usage information for each mobile subscriber and used this data mart to determine target subscriber groups. The team then designed and launched several below-the-line campaign waves based on best practices in the industry to the relevant customer groups. For example, some campaigns were designed to boost revenues by adjusting product offerings based on customer usage patterns by country.
Over the course of one year, the operator launched more than 250 targeted outreach campaigns, which achieved a 33-percent response rate, on average. The time to market for those campaigns dropped from 30 days to one week. And the biggest improvement was seen in customer interactions. Network traffic increased by 64 percent, daily active subscribers jumped by 17 percent, and revenues grew by 2 percent.
Is your data mature?
These results are not unusual for companies with a keen balance of data strategy and the customer experience. Yet many operators are at different points along the data maturity spectrum. In our experience, most operators fall into one of three data environments:
• Info-archive: Operators that have yet to start initiatives to leverage Big Data. They have limited or no analytics capabilities.
• Info-familiar: Those who have already embarked on the data journey but have not implemented a full-fledged solution. Any analytics they do have are ad-hoc and not coordinated.
• Info-smart: Operators that have created a robust Big Data environment. Customer information is aligned and shared across the business and advanced analytics are used.
At each point on the maturity spectrum, telecoms can identify their main Big Data issues and pain points, and then create a roadmap tailored to their right path of customer interaction evolution. Even info-smart companies can uncover new opportunities and areas of improvement. Projects within the roadmap can range from small, specialized quick-wins (broadband dashboard, international usage data mart, etc.) that address pressing business needs to major overhaul programs (data warehouse setup, business intelligence tool revamp, etc.) aiming at establishing key Big Data capabilities within the organization.
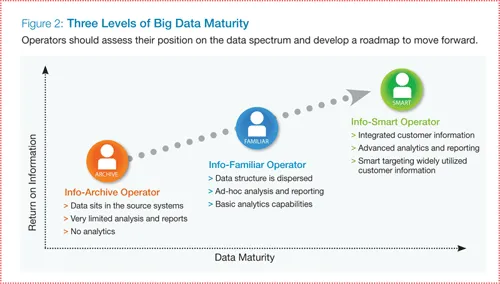
Regardless of maturity level, there are common issues that must be addressed by operators with developing Big Data strategy. Peppers & Rogers Group outlines some key recommendations across its OPIT framework:
Organization: One key requirement is to have a top-down governance team to direct all initiatives related to the overall data strategy of the organization. This requires a major cross-organizational effort to be led by a central team that owns the topic of Big Data. Members will define and prioritize Big Data strategy initiatives and work with teams from around the company to implement them.
Key lesson: The governance team must extend its primary responsibilities beyond just design to take a hands-on ownership role that actually drives progress and aligns different groups within the organization. This role cannot be underestimated.
Processes: Often, integrated operators have specific procedures and operations for each service line, making alignment across business units tricky. We recommend all departments follow the same methods for enterprisewide key tasks such as planning, target fixing and reporting.
Key lesson: Relationships between business and IT functions need to be streamlined and closely monitored. This enables the organization to transition from a silo structure where each department relies on its proper IT teams and procedures to a transversal approach that standardizes the IT function and the methods used to obtain, process, and analyze data.
Information: As with processes, there can be major challenges when each department's information is independent of one another. We have witnessed success when operators align the key moments of truth across different departments: the definitions of a new sale, a churner, or an inactive subscriber should be determined at the corporate level and aligned among different teams in charge of reporting and performance planning.
Key lesson: Often overlooked, it is vital to standardize definitions of KPIs, such as revenue, active subscribers' count, traffic, and ARPU. And we recommend that traditional KPIs such as voice, SMS and data usage be replaced by more granular KPIs that result from a thorough slicing and dicing of the existent transactional data.
Technology: Processing and analyzing Big Data is enabled by a wide variety of technical platforms. However, these technologies ought to complement Big Data strategies, not define them. Complex Event Processing (CEP), for example, provides powerful processing engines capable of analyzing in real time thousands of events issued by diverse source systems and short-listing actionable items based on a predefined set of rules. CEP systems are not independent architecture items, however. They must fit into the larger data architecture to be effective at driving relevant, consistent customer interactions.
Key lesson: More often than not, acting on technology is required, yet should be the last element of the data value chain to be considered.
When tasked with finding effective ways to use data, look to customer experience improvements. In both the short and the long term, operators can uncover new or better ways to interact with customers and deliver real ROI.